Blogginnlegg -
Wonders of Knowledge Representation
Knowledge representation is a field of science which you can find under the umbrella of artificial intelligence (AI). It is all about representing the world as we know it in a machine-readable manner. Describing facts and statements about our world, subject area and/or organization. Most commonly we represent knowledge using knowledge graphs, more precisely ontologies.
By: Veronika Heimsbakk, Knowledge Graph Lead, Insights & Data Nordics, Capgemini
What are Knowledge Graphs?
Graphs are all around us. They are all about reflecting reality in the data itself. It lets data flourish in its natural way of patterns and connectivity, and not forcing information into tables.
A knowledge graph is a unifying logical language for data. Equally interpretable by both humans, and machines, not depended on natural language. In a knowledge graph we represent knowledge as facts and statements, in ontologies.
An ontology is built up by triples denoted by global and unique identifiers. It holds accessible semantics for data—the meaning of things—directly in the data layer. Having the ability to capture the semantics of things, we can express knowledge directly in the data layer. With accessible semantics, we achieve capabilities as reasoning in data; to derive new insight from existing statements.
A visualized example of a knowledge graph
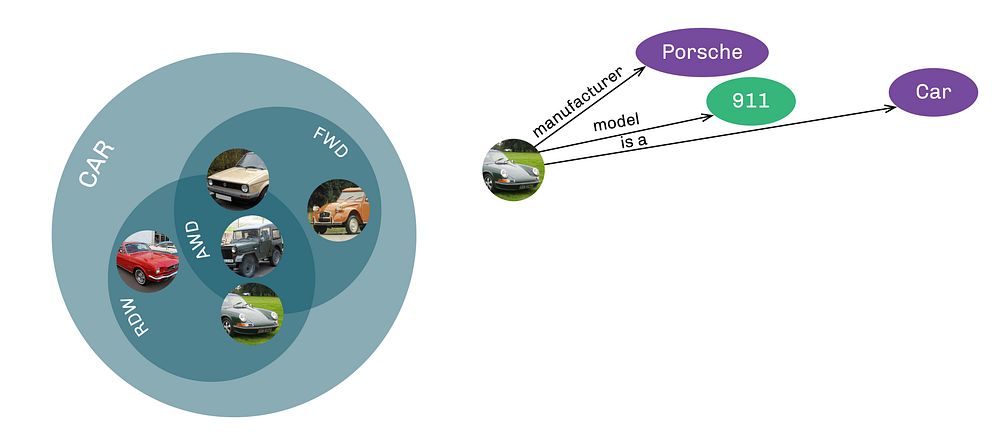
Knowledge graphs are all about expressing the knowledge of the world around us. Let us take a small part of the automotive domain as example. Let’s say I have a concept, Car. This concept is the abstract concept of Car, not the one physical car that I can touch. I can describe everything that a Car is, the semanticsof the concept car, by representing it in my knowledge graph.
The concept of Car can be divided into several sub-sets; read-wheel driven cars, front-wheel driven cars, and all-wheel drive cars. All this sub-sets of the larger set of cars holds elements, or instances if you like, the actual physical objects that you can touch. In this example we see one particular car that is a 911 model from the manufacturer Porsche. And by the way, this is exactly how the data is structured in the database!
Data attributes (literal values)
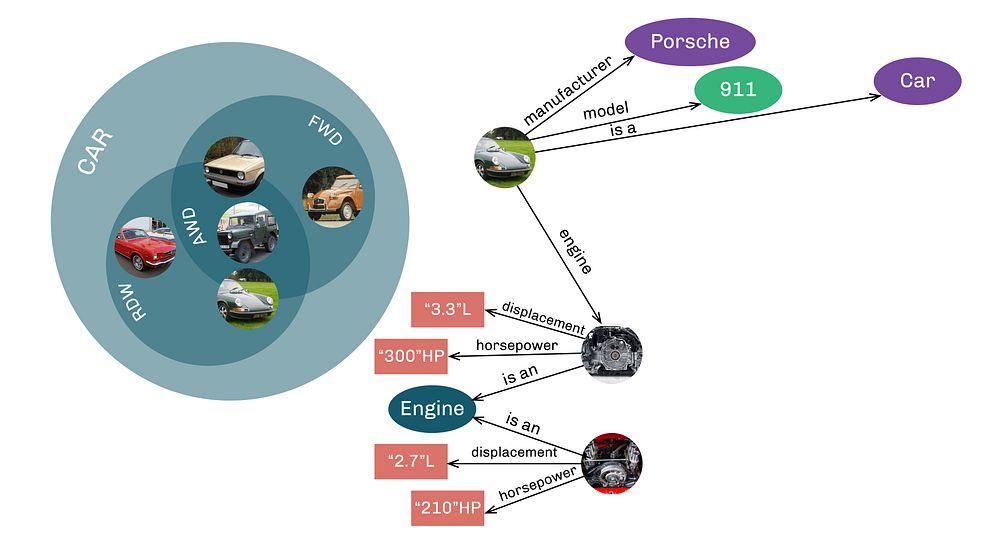
I can extend my graph by adding more concepts and instances to describe my car. My car has a particular engine that holds some technical specifications as data attributes. And these data attributes, they do not need to be double, float, int or something like that. We can type set data attributes in the actual unit! So, displacement in this case, is represented in liter.
A knowledge graph is a dynamic model
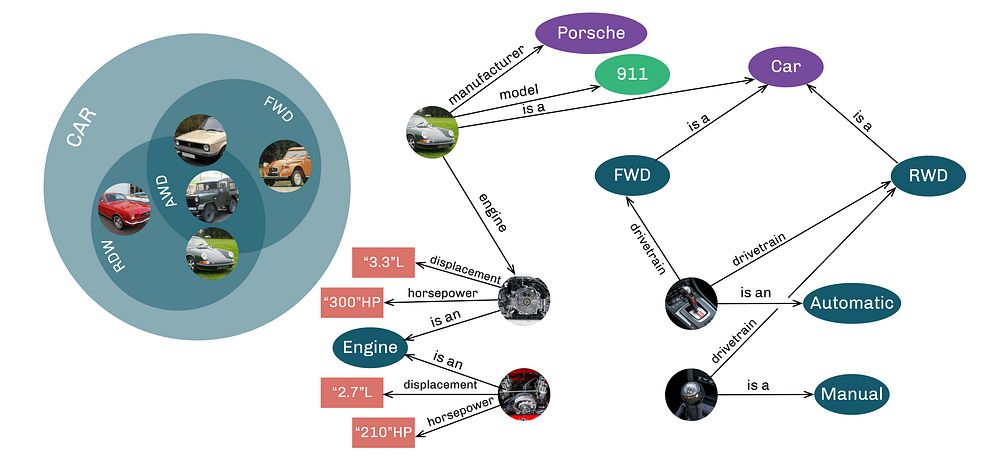
I can also further describe my abstract concepts, and add some more instances, like gearboxes. A knowledge graph is a dynamic model, ever expanding!
Connect to external ontologies to harvest knowledge!
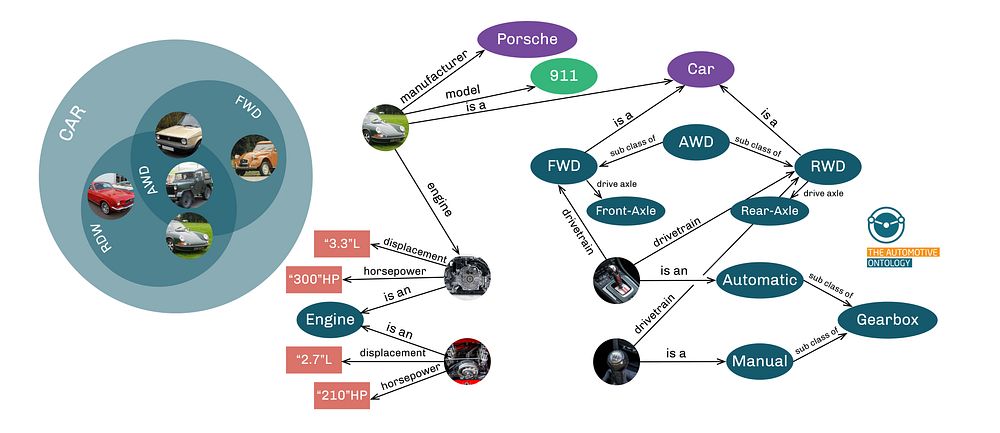
And since the automotive domain has been around for a few years, 138 years to be precise, there sure are global agreed upon definitions of the abstract concepts that makes up the automotive domain. In many cases, there exists domain ontologies available on the web, ready to be reused to enrich your data. I can harvest pre-defined definitions of well-known terms and concepts directly from The Automotive Ontology to enrich my knowledge graph of cars.
These external ontologies are developed by non-profit organizations, and other organizations. They are developed my researchers in academia, or by open-source communities. There are plenty of well-maintained ontologies waiting for you on the web.
Harvest instance data through open endpoints
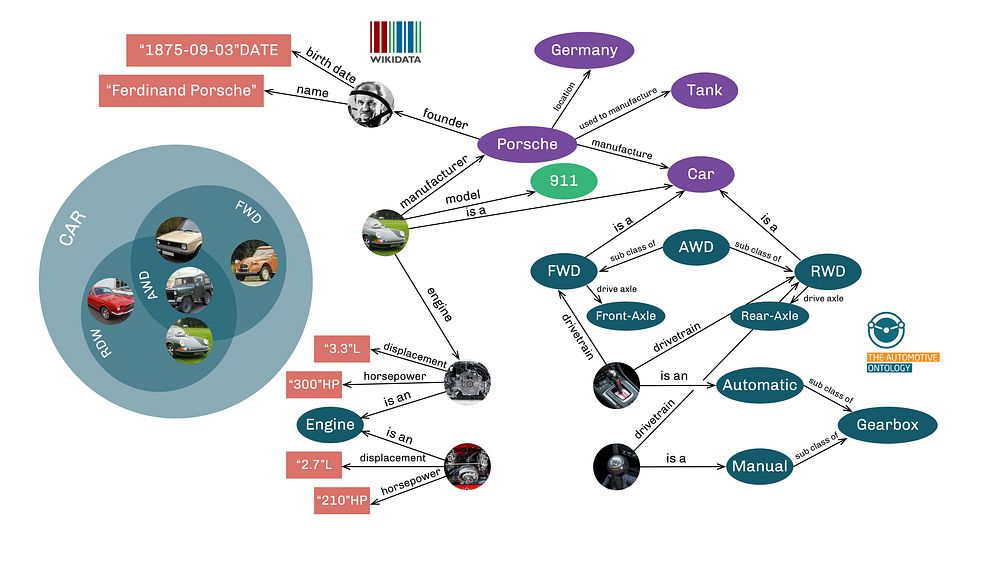
We can even harvest instance data from open endpoints as Wikidata! In the end, it is only your imagination, and maybe the boarders of your domain, who sets the limit for what you can express in a knowledge graph.
Reason your way to new knowledge!
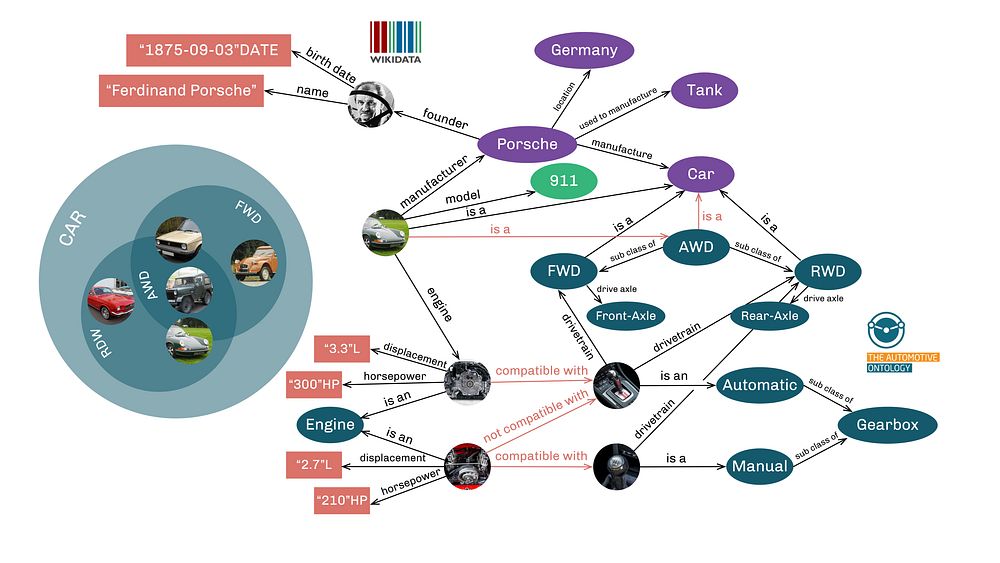
Imagine that my graph holds all technical specification connected to engines and gearboxes. And due to the restrictions of the specific engines and gearboxes, only a few pairs are compatible with each other. This notion of “compatible with” or “not compatible with” can then be reasoned by running a reasoning engine on the knowledge graph. And this without implementing ONE LINE of programming code! As almost every single triple store (graph database) and ontology tool supports this out of the box. A reasoner will add facts to your graph to fulfill any statement and fact that you have expressed for your world (read: domain). If there are any inconsistencies in your data, a reasoner will then discover a logical contradiction. If not, a whole new world of inferred facts might appear for you, providing new insight!
THIS is what differs knowledge graphs from most data-driven technologies. To be able to perform logical deduction in data. This capability is more important than ever in a world of huge amount of content, especially generated, probabilistic content.
Meet our knowledge graph experts at The Knowledge Graph Conference 2024
In Capgemini, we have a large team of knowledge graph experts all over the world. We find these technologies essential and crucial for achieving successful implementations within artificial intelligence. Therefore, Capgemini are proud bronze sponsors of The Knowledge Graph Conference this year. And we are representing with a team of four knowledge graph experts from all over Europe.
Don’t hesitate to reach out to any of us to learn more about Capgemini’s knowledge graph offerings and capabilities.
See you in New York?